What is Google Secure AI Framework?
A brief on Google Secure AI Framework (SAIF), a framework for ensuring AI models are secure-by-default when implemented.
As artificial intelligence (AI), and in particular generative AI, garners social mindshare and gets embedded into everyday technologies, concerns around trust, risk and security are growing rapidly. To help organizations understand and manage these concerns, Gartner has introduced the AI TRiSM framework, which I explored in my previous post. In this post, I'll cover another framework for deploying AI technologies in a responsible manner, this time published by Google - the Secure AI Framework.
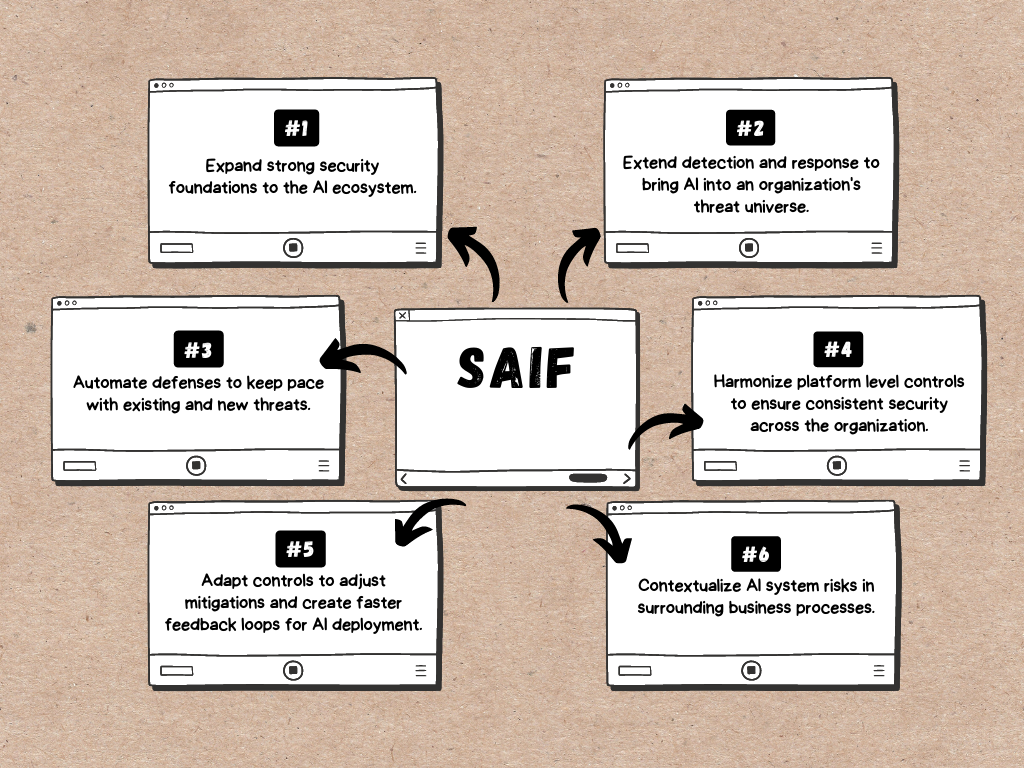
The Google Secure AI Framework (SAIF) is a conceptual framework for ensuring AI models are secure-by-default when implemented. Google is no stranger is security frameworks, especially those that appeal to the open-source community at large, like SLSA. According to Google:
SAIF is designed to help mitigate risks specific to AI systems like stealing the model, data poisoning of the training data, injecting malicious inputs through prompt injection, and extracting confidential information in the training data.
Before organizations use SAIF, they should understand the specific business problems AI will solve, the data required to train or fine-tune the models, and how the models interact with end users. This in turn drives the policies, protocols, and controls required to be implemented as part of SAIF. In particular, Google SAIF has six core elements:
- Expand strong security foundations to the AI ecosystem: this includes secure-by-default infrastructure, and organisational expertise that keeps abreast of evolving threat models. Existing security controls should be reviewed for applicability to AI systems and adapted as necessary; additional controls should be added to cover threat models unique to AI risks, and regulations. AI data governance is critical too, and all supply chain assets, code, and training data should be managed properly.
- Extend detection and response to bring AI into an organization's threat universe: this includes monitoring inputs and outputs of AI systems to detect anomalies, and the use of threat intelligence to anticipate attacks. Existing detection and response measures must be augmented to deal with attacks against AI systems. For generative AI use cases, output monitoring is crucial; malicious content creation, privacy violations, and general system abuse should be monitored and addressed.
- Automate defenses to keep pace with existing and new threats: this includes automation to improve the scale and speed of incident response. Generative AI can help automate time consuming tasks, reduce toil and fatigue, and speed up defensive efforts, but human oversight is necessary for important decisions to reduce bias, align on ethics, and improve fairness.
- Harmonize platform level controls to ensure consistent security across the organization: this includes consistency across control frameworks for risk mitigation across different platforms. Understanding AI model and application usage, building controls into the software development life cycle, and offering AI security risk awareness and training for employees is desired. Examine overlaps with existing security tooling and frameworks, and standardize on frameworks and controls to reduce fragmentation and inefficiencies.
- Adapt controls to adjust mitigations and create fast feedback loops for AI deployment: this includes continuous testing to understand the effectiveness of implemented controls, and adaptation to feedback. Novel attacks include prompt injection, data and model poisoning, sensitive data leakage, and evasion attacks, among others. Red team exercises can improve safety assurances, while continuous learning is critical for improving accuracy, reducing latency, and increasing efficiency of controls.
- Contexualize AI system risks in surrounding business processes: this includes end-to-end risk assessments that align with business processes and risks. Diverse groups and domain experts should be included to broaden perspectives, shared responsibilities should be understood when using third-party solutions and services, data privacy protections should be implemented, and AI use cases should be mapped to organisational risk tolerances.
In conclusion, Google SAIF is designed to help organizations raise the security bar and reduce overall risk when developing and deploying AI systems.