What is AI TRiSM?
A brief on AI TRiSM, Gartner's AI trust, risk and security management framework.
Artificial intelligence (AI) has become an integral part of our lives, from virtual assistants and chatbots to self-driving systems. Generative AI has only poured fuel into the proverbial fire, throwing several seemingly untouchable industries into disarray. According to a report from the McKinsey Global Institute, generative AI is set to add up to $4.4 trillion(!) in value to the global economy annually. However, as AI permeates our society through advanced technologies, concerns about AI trust, risk, and security, and their downstream implications on labor, economy, and our social fabric in general, have also grown rapidly. How do we ensure factual accuracy? How do we prevent plagiarism and intellectual property infringements? How do we reduce bias, align on ethics, and improve fairness?
While organizations move rapidly to create and capture value from AI, key stakeholders should consider the potential impact of AI trust, risk, and security in their decisions. Organizations that don't manage AI risks are far more likely to experience negative outcomes, failures and losses. According to Gartner:
By 2026, organizations that operationalize AI transparency, trust and security will see their AI models achieve a 50% result improvement in terms of adoption, business goals and user acceptance.
What is needed then is an approach to define and address these concerns in a systematic way. As is their wont, Gartner introduces another acronym AI TRiSM, short for AI (T)rust, (Ri)sk, and (S)ecurity (M)anagement, as "a framework that supports AI model governance, trustworthiness, fairness, reliability, robustness, efficacy and privacy. It includes solutions, techniques and processes for model interpretability and explainability, privacy, model operations and adversarial attack resistance for its customers and the enterprise".
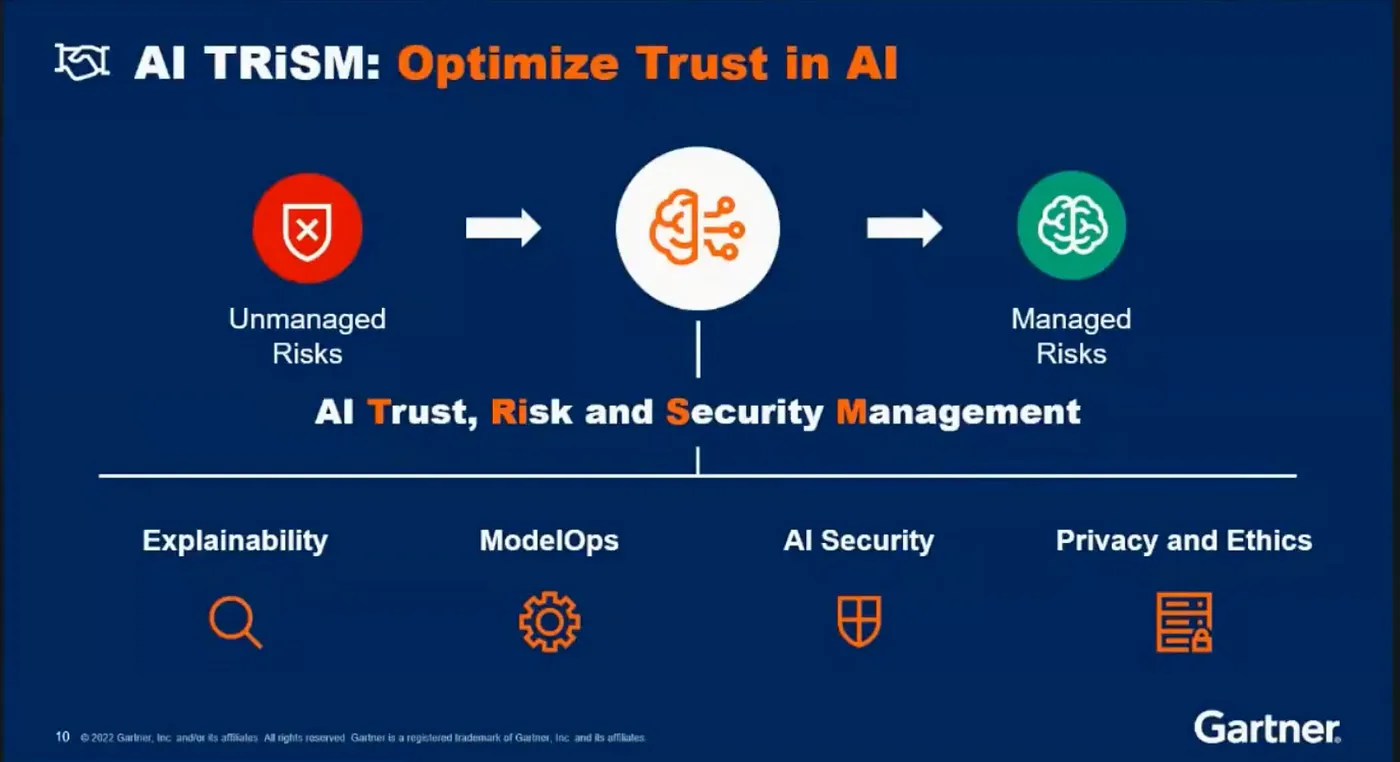
Gartner's AI TRiSM framework revolves around four key pillars:
- Explainability: refers to the ability of a model to provide clear and human-understandable explanations for its decision-making process. This is crucial for building trust with users, and for ensuring fairness and ethical outcomes.
- Model operations: involves management of the entire model lifecycle, from data preparation and ingestion, to model training, deployment and ongoing monitoring. This ensures the model is performing as expected, identifies and removes issues or biases, and continuously optimises its performance.
- AI security: critical concern for organisations, as evidenced by a growing class of adversarial machine learning attacks. The data and models are often extremely valuable, and robust security measures must be implemented to ensure their integrity and confidentiality.
- Privacy and ethics: ensures data is collected and used in a responsible and ethical manner, respecting the privacy of individuals along the way. Organisations must also consider the downstream socioeconomic impact of the usage of AI in their applications, and work to mitigate negative effects.
To achieve these objectives, organisations can consider the following items:
- Setting up of a dedicated task force to manage AI TRiSM efforts.
- Ensuring AI systems are designed in a way that preserves accountability.
- Implementing measures to manage AI systems and maximise business outcomes, rather than just meeting minimum compliance requirements.
- Including diverse groups and domain experts to broaden perspectives.
- Making AI models explainable or interpretable, and protecting the data used.
- Incorporating risk management into model operations by focusing on model and data integrity, and constantly validating them.